- See All Results For This Question
- Information Theory And Coding Questions And Answers Pdf
- Information Theory And Coding Vtu Notes Pdf
- Digital Communication Tutorial
- Digital Communication Resources
Information theory & coding (ECE) 1. INFORMATION THEORY AND CODING Nitin Mittal Head of Department Electronics and Communication Engineering Modern Institute of Engineering & Technology Mohri, Kurukshetra BHARAT PUBLICATIONS 135-A, Santpura Road, Yamuna Nagar - 135001. Information Theory and Coding Introduction – Definitions, Uncertainty, Measure/Properties of Information with Proofs ITC Lectures in Hindi for B.Tech, MCA, M.
- Selected Reading
Information is the source of a communication system, whether it is analog or digital. Information theory is a mathematical approach to the study of coding of information along with the quantification, storage, and communication of information.
Conditions of Occurrence of Events
If we consider an event, there are three conditions of occurrence.
If the event has not occurred, there is a condition of uncertainty.
If the event has just occurred, there is a condition of surprise.
Cim website of carmelito lauron. If the event has occurred, a time back, there is a condition of having some information.
These three events occur at different times. The difference in these conditions help us gain knowledge on the probabilities of the occurrence of events.
Entropy
When we observe the possibilities of the occurrence of an event, how surprising or uncertain it would be, it means that we are trying to have an idea on the average content of the information from the source of the event.
Entropy can be defined as a measure of the average information content per source symbol. Claude Shannon, the “father of the Information Theory”, provided a formula for it as −
$$H = - sum_{i} p_i log_{b}p_i$$
Where pi is the probability of the occurrence of character number i from a given stream of characters and b is the base of the algorithm used. Hence, this is also called as Shannon’s Entropy.
The amount of uncertainty remaining about the channel input after observing the channel output, is called as Conditional Entropy. It is denoted by $H(x mid y)$
Mutual Information
Let us consider a channel whose output is Y and input is X
Let the entropy for prior uncertainty be X = H(x)

(This is assumed before the input is applied)
To know about the uncertainty of the output, after the input is applied, let us consider Conditional Entropy, given that Y = yk
See All Results For This Question
$$Hleft ( xmid y_k right ) = sum_{j = 0}^{j - 1}pleft ( x_j mid y_k right )log_{2}left [ frac{1}{p(x_j mid y_k)} right ]$$

This is a random variable for $H(X mid y = y_0) : .. : .. : .. : .. : .. : H(X mid y = y_k)$ with probabilities $p(y_0) : .. : .. : .. : .. : p(y_{k-1)}$ respectively.
The mean value of $H(X mid y = y_k)$ for output alphabet y is −
$Hleft ( Xmid Y right ) = displaystylesumlimits_{k = 0}^{k - 1}Hleft ( X mid y=y_k right )pleft ( y_k right )$
$= displaystylesumlimits_{k = 0}^{k - 1} displaystylesumlimits_{j = 0}^{j - 1}pleft (x_j mid y_k right )pleft ( y_k right )log_{2}left [ frac{1}{pleft ( x_j mid y_k right )} right ]$
$= displaystylesumlimits_{k = 0}^{k - 1} displaystylesumlimits_{j = 0}^{j - 1}pleft (x_j ,y_k right )log_{2}left [ frac{1}{pleft ( x_j mid y_k right )} right ]$
Now, considering both the uncertainty conditions (before and after applying the inputs), we come to know that the difference, i.e. $H(x) - H(x mid y)$ must represent the uncertainty about the channel input that is resolved by observing the channel output.
This is called as the Mutual Information of the channel. Media player 12 for windows 7 download freesoftrareabcsoft.
Denoting the Mutual Information as $I(x;y)$, we can write the whole thing in an equation, as follows
$$I(x;y) = H(x) - H(x mid y)$$
Hence, this is the equational representation of Mutual Information.
M4V (iTunes Video) is a container for audio, video (H.264, AAC, and Dolby Digital) from Apple, supports DRM copyright protection. MP4 (MPEG-4 Video) is a multimedia container and a compression. Convert M4V to MP4 with free M4V converter. Convert videos, movies, audio clips, and media recordings from Apple iTunes to MP4 format. Keep the original HD quality, 5.1 audio, and subtitles. Watch your favorite MP4 media files on any device: mobile phones, tablets, TV, PC, DVD players! Download best M4V to MP4. How to Convert M4V to MP4? Click the “Choose Files” button to select your M4V files. Click the “Convert to MP4” button to start the conversion. When the status change to “Done” click the “Download MP4”. 3 Recommended Converter Tools for M4V to MP4 Conversion. It probably be hard for you to watch M4V file on Android phones, Windows PC, PSP, Xbox or edit the M4V video in iMovie, Final Cut Pro, since the M4V. M4v to mp4 converter downloadsarah smith.
Properties of Mutual information
Information Theory And Coding Questions And Answers Pdf
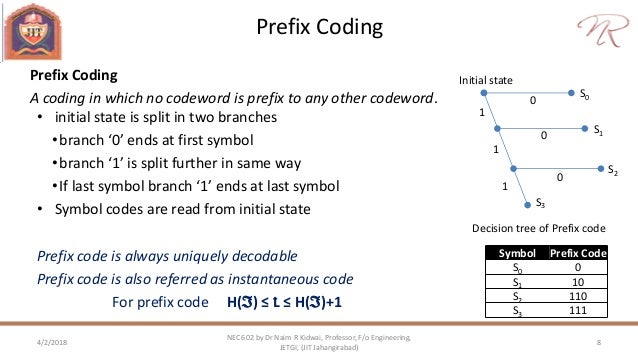
These are the properties of Mutual information.
Mutual information of a channel is symmetric.
$$I(x;y) = I(y;x)$$
Mutual information is non-negative.
$$I(x;y) geq 0$$
Mutual information can be expressed in terms of entropy of the channel output.
$$I(x;y) = H(y) - H(y mid x)$$
Where $H(y mid x)$ is a conditional entropy
Mutual information of a channel is related to the joint entropy of the channel input and the channel output.
$$I(x;y) = H(x)+H(y) - H(x,y)$$
Where the joint entropy $H(x,y)$ is defined by
$$H(x,y) = displaystylesumlimits_{j=0}^{j-1} displaystylesumlimits_{k=0}^{k-1}p(x_j,y_k)log_{2} left ( frac{1}{pleft ( x_i,y_k right )} right )$$
Channel Capacity
Information Theory And Coding Vtu Notes Pdf
We have so far discussed mutual information. The maximum average mutual information, in an instant of a signaling interval, when transmitted by a discrete memoryless channel, the probabilities of the rate of maximum reliable transmission of data, can be understood as the channel capacity.
It is denoted by C and is measured in bits per channel use.
Discrete Memoryless Source
A source from which the data is being emitted at successive intervals, which is independent of previous values, can be termed as discrete memoryless source.
This source is discrete as it is not considered for a continuous time interval, but at discrete time intervals. This source is memoryless as it is fresh at each instant of time, without considering the previous values.
